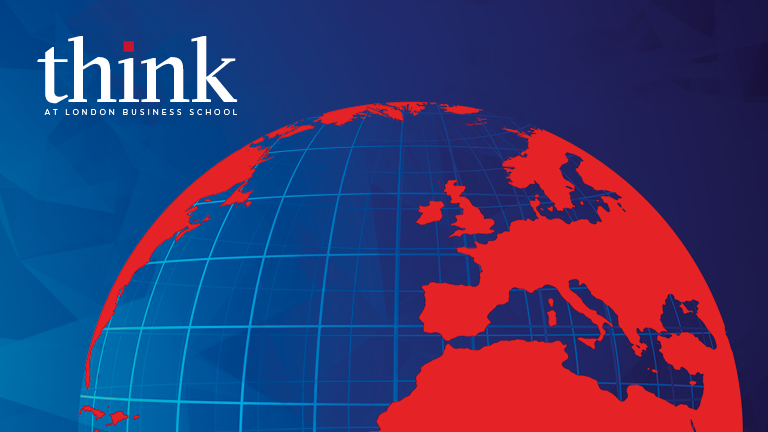
Discover fresh perspectives and research insights from LBS
Think at London Business School: fresh ideas and opinions from LBS faculty and other experts direct to your inbox
Sign upPlease enter a keyword and click the arrow to search the site
Or explore one of the areas below
To unleash automation’s decision-making potential we must examine its limitations
In the not-too-distant past, we made decisions based entirely on human judgement. Now, automated systems are helping people call important shots. Financial institutions use algorithms to offer loan applicants immediate yes-no decisions. Recruitment firms adopt systems powered by language technology to match applicants to vacancies. Even the criminal justice system uses predictive algorithms when sentencing criminals.
There are hundreds of examples like these, and algorithmic automation of decision-making is only set to rise. Why? First, computational power is becoming cheaper thanks to Moore’s “Law” – the observation that the number of transistors per square inch in integrated chips, a measure that correlates with computation power, doubles every 18 months. Second, we’re creating smarter algorithms that can transform raw and unstructured data into digestible information that is impacting everything from digital to financial health. Third, we simply have more data. Every aspect of our lives is blazing a digital trail which can be mined to better understand human behaviour – and predict the future.
Some people argue that algorithms can never match human ability in making decisions because they focus too narrowly on specific tasks. But are humans so perfect? People can be influenced by how they feel: one study of more than a thousand court decisions showed that judges are more lenient after lunch. People can be slow: JP Morgan Chase cut 360,000 hours of routine finance work to a matter of seconds with a system that stopped 12,000 mistakes made by human error every year. People can be selfish: research led by Madan Pillutla, London Business School Term Chair Professor of Organisational Behaviour at London Business School, suggests that even the fairest, most well-intentioned person can be prone to discriminate – they hire based on what’s in it for them.
It’s true that algorithms do not suffer from human imperfections, such as being tired, error-prone or selfish. But there are limitations when we rely on algorithms to make decisions, and it’s important we understand what they are:
1. Transparency: algorithms are a black box – it’s difficult to know if they’re fit for purpose if we don’t know how they work
2. Bias: algorithms are trained to make recommendations based on data that’s not always representative – systematic biases can go unnoticed and these biases can proliferate over time
3. Accuracy: we treat algorithms as infallible – in reality, they’re only designed to work well on average.
An example from the criminal justice system can help us examine these limitations in action. Eric Loomis was arrested in the US in February 2013 and was accused of driving a car that had been used in a shooting: he pleaded guilty to eluding a police officer. The judge at Wisconsin Supreme Court sentenced him to six years in prison. Part of the decision-making process was COMPAS (which stands for Correctional Offender Management Profiling for Alternative Sanctions), a proprietary algorithm used to calculate the likelihood that someone will reoffend. COMPAS measures categories such as criminal personality, substance abuse and social isolation. Defendants are ranked from 1 (low risk) to 10 (high risk) in each category.
Loomis challenged the judge’s use of the COMPAS score because unlike other evidence used against him, his defence team could not scrutinise the algorithm – the first limitation. The judge’s reliance on the score, the factors considered and the weight given to data in the decision-making process were all grounds for his appeal, which reached the Wisconsin Supreme Court in July 2016.
The second limitation, that algorithms are only as good as the data used to train them, is also an issue. COMPAS uses crime data, which is essentially arrest data. Arrest data often relies on police being in the right place at the right time. If an area is notorious for petty crime, police are more likely to attend, make an arrest and report the data. The information is then used to predict future crimes. The problem? At some point, this becomes self-perpetuating. What if a crime goes unreported because a neighbourhood has a reputation for upholding the law? What if the police simply aren’t able to attend? No police, no arrests, no data.
Think at London Business School: fresh ideas and opinions from LBS faculty and other experts direct to your inbox
Sign up