AI for global good
Toby Velte explains three ways to partner with AI to supercharge your global initiatives
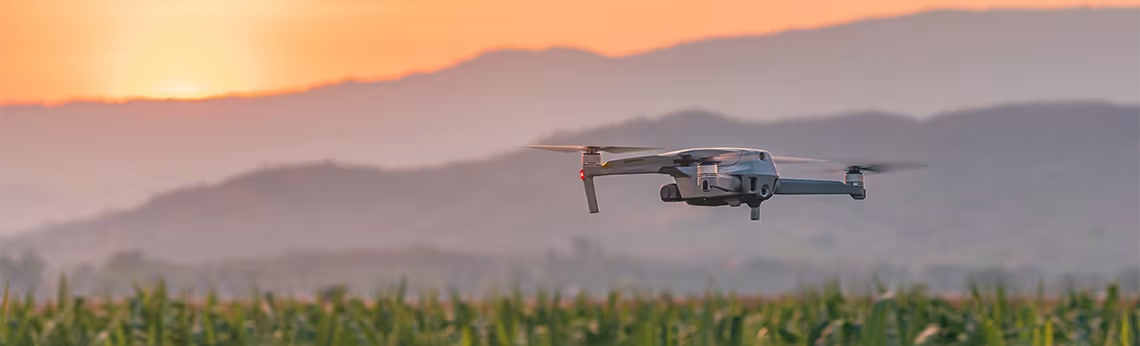
There is tremendous hype today about how AI will 'change the world.' However, it's not apparent from the press and media how this will actually take place or how it will impact us, other than almost certainly taking our jobs. Technology leaders are jumping aboard, fanning the hype flames of AI being – on one hand – as important as electricity (Google CEO Sundar Pichai, 2018) or one of the biggest threats to humanity (Elon Musk, 2023).
I’m in the code of AI every day and have a more optimistic view. Since AI first left the confines of academia, the business entity has often been the vehicle for using AI for good. Generative AI (GenAI) has the attention of the media now, but is only a fraction of AI that can work on our behalf. So, I am going to focus here on the larger set of AI tools (okay, I’ll use a little generative AI) to illustrate how AI can have a profound impact on some of the most daunting global challenges we face. Let’s jump in…
The trick is to ask the right question then select the best machine learning tools and data to apply to that question. Doing this accurately requires, what I call, AI intuition. It involves ethical considerations, sustainable methods, and technical and business depth. As you’ll see in the examples below, AI tends to be applied to high-value situations first. In agriculture, for example, high-revenue plants such as medicinal cannabis and ornamental flowers are the target of AI. In medicine, neurosurgery and common diseases are tackled first, and in individual performance, we started with professional athletes. As AI becomes more commonly applied, it will trickle down into all aspects of that domain.
Discover fresh perspectives and research insights from LBS
'A robot can scan hundreds of plants every hour and send its data up to the cloud for storage and AI analysis'
1 AI-Powered robotic agriculture
Feeding the planet while reducing our impact on energy, water, and land resources is a key driver in ag-tech. But how much more optimisation can we really have on yields and resource consumption? Low-cost robotics using 3D-printed enclosures chock full of inexpensive sensors can scan plants indoors on their grow racks or outdoors using drones fitted with this technology (budscout.ai). These robots can get ‘plant intimate’ and capture millions of data points about the plants and growing conditions including light sources, infrared light reflectance off the plants, and air metrics like CO2 concentrations and temperature. Cameras can take images of growing plants throughout their lifecycle. For an initial cost of a few hundred pounds, a robot can scan hundreds of plants every hour and send its data up to the cloud for storage and AI analysis.
Robots indicate when there is an issue a grower needs to address or when to perform certain growing functions, such as plant trimming. The robot scans the plants in real time taking images. AI uses these images to determine the species of plant and specific cultivars. Infrared readings of the temperature of the plant leaves versus the ambient temperature can indicate how effectively the plants are using and storing water. AI can use object detection to identify what is being grown (such as tomatoes or a flower) or things that shouldn’t be there, such as pests. Each tomato in this example could be tracked over its total growth to harvest. Knowing the entire crop number and size of the product is useful for crop insurance and forecasting yield well in advance of harvest.
If an issue is detected, a grower can send a technician to the plant to remedy the issue before there is a significant impact on yield for that plant. In our laboratory studies we found an increase in plant yield of ~25% when the AI spotted an issue and sent a worker to intervene over when the grower could see an issue visually and intervene. With a shortage of agriculture workers, they are sent out only to fix issues, not to look over thousands of square metres of plants in the hope of spotting a problem.
This plethora of data is also used to optimise the amount of generated light they receive for indoor grows and water intake. Shutting down lights and restricting water if no longer needed by the plants would dramatically reduce the consumption of these resources. The robots themselves can be powered by solar energy stored in a battery on the device. Indoor growing consumes massive amounts of electricity for lighting and is a grower’s main expense, so even a small reduction of light use goes a long way to reduce energy consumption and improve operating costs.
'There is a growing call from neurosurgeons and caregivers to use technology such as AI '
2 Extending healthcare everywhere
Globally, there is a massive dearth of qualified neurosurgeons in spite of neurological issues being a major cause of death and disability. The need is growing with an ageing population, and in many low-and medium GDP parts of the world, the training and setup costs can never be recouped. There is a growing call from neurosurgeons and caregivers to use technology such as AI to make neurosurgery more accessible and safer globally. It is, of course, a model for all healthcare challenges to increase access, lower costs, and improve outcomes.
We are not ready to make a solution that immediately jumps to robotic neurosurgery. This would be a risky proposition fraught with safety, legal, and technical hurdles to overcome. So instead, large AI projects like this can be phased in to bring value quickly and reduce risk while building towards the goal of providing neurosurgery where it doesn’t exist today.
In this example we’ll start by creating a predictive machine learning model that can accurately predict the outcomes for patients prior to a proposed neurosurgery. We’ll need data – lots of data, because we need very high levels of accuracy. We can start with brain scans which are a set of images taken at various levels of a patient’s brain. Stacked together this forms a 3-D volume or voxel. This way we can see the tumour as it exists in the patient. We couple this to many other forms of data such as patient demographics, physiological measures, and outcomes post-surgery.. We use this to train a new AI model to make predictions about outcomes for prospective patients. Neurosurgeons use this to make recommendations to the patient now that they better understand the likelihood of possible adverse events. At this stage, we are simply providing the surgeon with new insights. They are still making 100% of the decisions along with the patient.
In subsequent stages, we’ll address one of the main challenges for neurosurgeons which is selecting the best pathway to the tumour. This is done to avoid possible damage to critical areas and pathways in the brain such as those used for speech and motor control. While we may have thousands of brain scan volumes available to use showing real patient tumour locations, we need many more to create an AI model to suggest the optimal path. Here’s where GenAI comes in.
Before people started creating images of skateboarding cats on the moon, one of the initial uses of GenAI was to create synthetic data for machine learning training models. We’ll use what is called a Generative Adversarial Network (GAN). It is two AI models pitted against each other. First, the discriminator is trained up on thousands of existing brain voxels to recognise brains with tumours in them. Next the other model called the generator tries to create a new brain voxel without knowing anything about what it is supposed to look like. It sends its attempt to the discriminator which can accept or reject the image as good enough.
At first the generator just makes images full of static noise which are summarily rejected. Over time it gets more parts correct and through millions of iterations can create convincing new brain scan images. Now armed with millions of brain voxels, other AI models are trained to select the best pathway to take. The most ambitious phases would use these models to ultimately control a robotic end effector to perform surgeries.
3 Dynamic and optimised team building
In situations where human performance is critical, AI is being used to select the optimal individuals and teams to perform those critical jobs. Today, machine learning is accurately predicting the future performance and availability of professional athletes (probility.ai) in American football (NFL) and hockey (NHL). Using only publicly available data and looking at past performances, injuries, and other information for athletes over the past two decades, it is possible to train an AI model that predicts (with usable confidence) future injuries and performance for active athletes.
For example, by training an NHL model on all professional hockey players that have had their whole career contained within the past 20 years, we can predict total games a current player will have in their career. This prediction is not perfect but comes with an R-squared value of 0.82 which can be quite useful in selecting players for your roster and constructing their contracts. The level of confidence in the AI predictions needs to match the usefulness and risk factors. This is not neurosurgery and the current predictive power used by coaches, etcetera, is extremely low so an R-squared value of 0.82 is useful and matches the risk factor.
'Finding the ideal team in the moment to maximise the likelihood of positive outcomes could soon become a reality'
These data and insights can also be useful from other perspectives. From a player’s view, knowing that your career might be cut short due to injury would have a significant impact on how you may play (subsequently changing the player’s future). It could, however, also be used to better understand what about the player is influencing the prediction. If we can understand this, we might be able to change it. Some features of the player such as their weight and what team they play on are changeable. We know that when we make these hypothetical changes and run the player through the model again, we get a different prediction. Some features, including a player’s age and height are not as plastic. This highlights the importance of ethics in AI in deciding what predictions to make and how these insights are presented and to whom.
Predictions with similar levels of confidence can be made today for other career metrics such as total goals scored or assists. They can also predict next season metrics such as playing time and total number of injuries. It’s just a matter of data collecting, cleaning, and model building before this is carried out for more immediate time frames (next game or next play) and other sports and scenarios other than professional sports. Fire fighters, police officers, surgeons, negotiation teams, board of directors, and more could all be selected based on predicted performance. Moreover, by looking at immediate physiological responses from individuals, we may be able to determine how well each will perform in the moment. Getting a poor night’s sleep, struggling with anxiety from personal conflict, or fatigue from a strenuous workout could all hinder an individual’s potential.
Team performance is much more than the collective sum of individuals on the team – there is something special when team dynamics are present. Work is underway now to assess and predict how different individuals come together in a team setting for optimal group outcomes. It is not simply the grouping of the most talented (and expensive) individuals to guarantee the championship or best board dynamics. It turns out that in professional sports, different athletes have different outcomes when matched with different teams (and coaching staffs, etcetera) and presumably with different team members. Dynamic teams of leaders and those with specialised skills can be pulled together globally in a moment’s notice to tackle some of the most prominent issues. Finding the ideal team in the moment to maximise the likelihood of positive outcomes could soon become a reality.
Unlike training a new Large Language Model such as ChatGPT, these examples can be generated quickly and cheaply. It’s a relatively straightforward matter to tackle different agricultural needs, disease states, or team dynamics. With no code options and low-cost computing available, the barriers have never been lower to use AI. Now is the time to ask your own challenging questions and build up your AI intuition.
Toby Velte is the co-founder and chairman of Budscout.ai and Probility.ai and a 2024 Sloan Fellow at the London Business School.