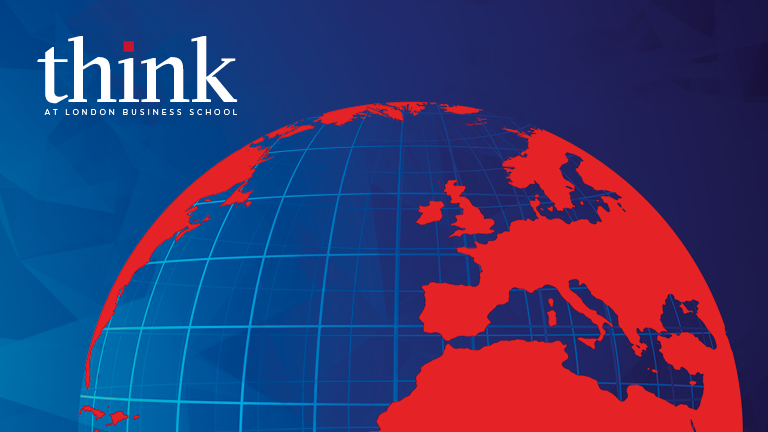
Discover fresh perspectives and research insights from LBS
Think at London Business School: fresh ideas and opinions from LBS faculty and other experts direct to your inbox
Sign upPlease enter a keyword and click the arrow to search the site
Or explore one of the areas below
Machine learning is opening up new avenues in behavioural finance – but human curiosity and intelligence are still essential
With rational market theories largely debunked, applying sophisticated strategies for managing behavioural biases is critical for investors to gain the upper hand over their peers. Machine learning has become an increasingly significant tool with potential for it to enhance performance or even stabilise markets.
Anna Pavlova, Professor of Finance at London Business School and Academic Director of the AQR Asset Management Institute, says that, while the field of behavioural finance is well-established and widely applied in practice, there is still a great deal of scope for further research: “As machine learning and big data become ever more part of the industry we would particularly like to see further research in the area of de-biasing that could really benefit practitioners in improving their decision-making ability by avoiding behavioural biases.”
Behavioural finance theory has identified three main types of bias, which can explain many central facts about markets, including average returns, time series, predictability, momentum and reversals, bubbles, and trading volume:
1.We tend to over-extrapolate the past, putting too much weight on the recent past (such as recent performance) while making decisions and forming beliefs about the future.
2.We tend to be over-confident in our own ability over other people’s, as well as in the accuracy of our beliefs. According to Nicholas Barberis, Stephen and Camille Schramm Professor of Finance at Yale School of Management, “The irrationally high trading volume we observe in financial markets is one piece of evidence for over-confidence in our own ability where those on both sides of a trade have to believe they have superior knowledge of the future prospects of the asset than the other.”
3. We tend to be more sensitive to the prospect of loss over gain, and to give too much weight to low-probability outcomes.
Added to these is another bias, according to Nobel Laureate Robert Shiller, Sterling Professor of Economics at Yale University, the influence of narratives and their contagion. As he comments, “The current biggest influencer on the US stock market is the narrative of Donald Trump as cutter of taxes and regulations giving a boost to earnings, despite a rational understanding being that these effects will only be temporary.”
Research into the effects of narrative was simply not possible before the existence of big data. There is now huge potential to demonstrate how narratives might be affecting the fluctuations of the market, he suggests – and, once we are aware of this, we might be able to mitigate these effects.
Madan Pillutla, Professor of Organisational Behaviour at London Business School, outlines four main areas of existing research: incentives; training and education; choice architecture, often referred to as nudge; and frugal heuristics. Studies have shown that the first three may have limited impact on behaviour.
Professor Pillutla sees the last of these as providing the most potential for developing ways of de-biasing investor behaviour. In theory, through understanding better how information can be presented it might be possible to align it with the way the brain has evolved to process information and thereby encourage the brain to make more effective decisions.
On-the-ground market practitioners use behavioural finance approaches to attempt to maintain a performance edge. JP Morgan Asset Management has had a behavioural finance team in place for more than 20 years. “The key to our success has been to structure our investment decision-making process in a way that tries to prevent people allowing emotions to lead to biases,” comments Michael Barakos, Managing Director.
His firm attempts to make decisions based only on information that is empirically proven and tries to block out the majority of information, which can be classified as “noise”. It does this by combining a quantitative or computer modelling approach with an expert fundamental approach.
Similarly, Fidelity uses modern data analysis techniques to quantify some of what might be influencing the investment team emotionally. They also use carefully designed ‘nudges’ that potentially counteract portfolio managers’ instincts to trade on emotions and to succumb to the confirmation bias, a tendency to focus on evidence that corroborates their own views. The nudges flag up interesting phenomena in the markets that the managers may have overlooked with the aim of keeping their mental options open. They work on the basis that people’s decisions are made through a combination of the rational and emotional, and the main thing that can go wrong is when people stop thinking about all the options.
According to Barney Rowe, Senior Data Scientist at Fidelity, “Mental diversity and the ability to use behavioural investment techniques is what can give active managers the upper hand in a bear market so the aim of the Fidelity team is to encourage doubt where it could be useful and also curiosity where that may be lacking.”
According to Marcos López de Prado, Principal, Head of Machine Learning, AQR Capital Management, machine learning has multiple applications in behavioural finance that can help investors both profit from competitors’ biases and protect them from their own biases. Firms are increasingly using textual sentiment analysis to model overconfidence of market participants and meta-labelling to help size bets, and identifying exponential patterns to monitor or respond to greed and fear. The Flash Crash of May 2010 is a perfect example of the latter being put into action, with unbiased machines managing to stabilise the market shortly after humans panicked.
López de Prado explains, “Human fear had driven the initial nosedive, and after about three minutes all market makers were gone. Then with markets down 10%, the rational machines (“high frequency traders”) kicked in and stemmed losses.”
In the UK, the Financial Conduct Authority’s (FCA) primary concern in behavioural biases is not how to enhance performance. Rather, it is how the end consumer tends to overlook charges when purchasing financial products and how to encourage them to make more informed choices. Charges can make an enormous difference to returns over the long term but retail investors consistently fail to take them into account when deciding on a financial product, according to the FCA’s research using click-stream data, in collaboration with the Chicago Booth School of Business.
Explanations for this failure include limited attention beyond the information presented in marketing material, which usually focuses on past performance, but also a lack of understanding of the effect of compound interest on returns over the long-term. The FCA has looked at various avenues to improve understanding and wants to work increasingly with the investment management community through sharing data and ideas to improve communication to consumers.
But big data can’t do everything – so some consultancies, such as Towers Watson, still prefer qualitative rather than quantitative data in its assessment processes. Luba Nikulina, Global Head of Manager Research at Towers Watson, explains that it focuses heavily on the importance of organisational culture when advising clients on where to allocate their assets.
“When we are assessing culture we define it as the collective influence from shared values and beliefs on the way the organisation thinks and behaves,” she says. “The framework is divided into three: the first being how the organisation delivers value to its clients, the second being how it attracts, retains, and develops its personnel, and the last, most important one, is leadership.”
Adrian Furnham, Professor of Psychology at University College London (UCL) and Principal, Behavioural Psychology at Stamford Associates, additionally describes the individual characteristics that make a good portfolio manager. He highlights curiosity as the single best predictor of intelligence and survival for an investor, alongside quirkiness, adaptability, flexibility, honesty and integrity.
NN Investment Partners also uses big data to study behavioural bias and demonstrate to their portfolio managers where their biases are coming into play, the awareness of which will help them make better decisions. The basic structure of the investment process remains extremely important. An obvious example where diversity of thought can go awry is if a more senior person shares their opinion early on in the decision-making process and overly influences the opinion of those that follow.
A good feedback and review process in order for investors to learn from previous decisions is key, says Ewout Van Schaick, Head of Multi Asset at NN Investment Partners. He explains, “Behavioural science has become in a lot of ways a quantitative science. We try to always combine fundamental analysis techniques with our own human intelligence. Put simply, we combine man and machine.”
The AQR Asset Management Institute’s Insight Summit on Addressing Behavioural Biases in Investing brought together leading experts from academia and industry to discuss the latest insights and trends.
Think at London Business School: fresh ideas and opinions from LBS faculty and other experts direct to your inbox
Sign up