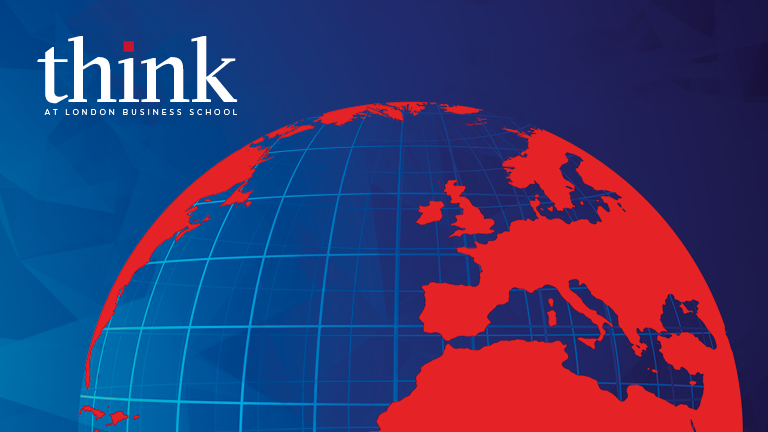
Discover fresh perspectives and research insights from LBS
Think at London Business School: fresh ideas and opinions from LBS faculty and other experts direct to your inbox
Sign upPlease enter a keyword and click the arrow to search the site
Or explore one of the areas below
Investment in data science can reap great rewards. If it doesn’t yield what you hope for, ask where you’re going wrong
As the behavioural economist Dan Ariely once tweeted, “Big data is like teenage sex: everyone talks about it, nobody really knows how to do it, everyone thinks everyone else is doing it, so everyone claims they are doing it.”
He made a good point. Grasping the potential of big data, recognising its limitations and seeing how it can be harnessed are not easy. Maybe your company has been exploring the use of big data for a while and now you’re wondering why it hasn’t revolutionised the way you interact with your customers or transformed your internal processes. Maybe you even hoped it would revolutionise your whole business model, and yet it hasn’t. You would be in good company. There is a gap between companies’ expectations of data science and what data science can actually do.
It’s not that big data itself is failing. Big data is just a set of methods (see box below). But how can you set up the data science teams to harness the power of big data for you? The success or failure of your data science team doesn’t depend on information systems or computational power. Data and infrastructure help but, in our experience, it’s rarely the reason why data scientists fail to instigate fundamental change and create value. More often it’s because their skills aren’t being properly used. Here are four common mistakes.
1. You give your data scientists the wrong problem to work on. Your organisation is asking questions that are impossible to answer; or you are demanding a solution to a problem that’s too complex to solve with clever data analysis alone. What’s at fault here is the starting point, the input to the data science “process” – so don’t be surprised if you don’t get the output you want. You’re wasting resources, missing opportunities and undermining trust in the data science team’s ability to improve business performance.
Learning the lesson: An online retailer asked an analyst in my team to provide evidence to support a business hunch that shorter delivery times would increase the conversion rate (the number of people who bought the product after viewing it). The analyst couldn’t find supportive evidence but, instead of accepting that delivery times were not the most important conversion factor, the client asked him to approach it in different ways to try to come up with the evidence it wanted.
2. The data scientists are working on the right problem but in the wrong way. Often data scientists get excited about finding novel solutions that push the boundaries of what’s possible. When they work in a corporate environment, they can become carried away and end up engineering solutions that are unnecessarily complex or too sophisticated for the problem at hand. This makes the solutions expensive and more difficult to implement – and as a result they have less impact. Choosing the right solutions – solutions that are sufficiently simple but not simplistic - requires solid understanding of the business context.
Learning the lesson: I worked on a project that tried to bring together data from several different places into one place. After a year, and with the support of those supplying the multiple databases, the completely redesigned architecture was still too unstable to use for customer-facing applications. We had been too ambitious. Our solution was over-engineered. Simpler relational databases would have allowed us to use existing skills and capabilities without compromising the customer experience.
Learning the lesson: When working for a large UK retailer, my team built a data-driven simulation of a new distribution centre. We were asked to develop complex error-correcting algorithms to account for inaccuracies in historical data and arrive at better precision. However, correcting historical errors would not have yielded greater accuracy as there were other, more material, ways in which our data was wrong. For example, at that time we were not even sure what products the distribution centre would stock.
3. Solutions are offered – but ignored. The rest of the organisation consistently ignores the work of the data scientists, failing to follow their recommendations or fully implement their algorithms. Sometimes this is because internal politics get in the way. It may be that internal stakeholders are too busy firefighting to listen to what the data scientists are saying. The data scientists can be at fault here too. Perhaps they’ve alienated their non-technical colleagues by emphasising the algorithm’s complexity and cleverness instead of how it can help make their daily lives easier. Perhaps some stakeholders even feel threatened, as if the data science team is designing solutions that are taking away an important part of what they do for the firm.
Learning the lesson: My team had had created a price-optimisation algorithm for general merchandise products such as small domestic appliances. In our tests, our algorithm improved profits significantly compared with the outcome when prices were set by the buyers. Nevertheless, we found significant resistance to rolling out the algorithm across categories. Many buyers simply did not want to use it. Those who did use it tended to be the most time-constrained. They saw the algorithm as something that would save them time and allow them to devote their efforts to other aspects of their job and therefore deliver more value to the organisation.
Learning the lesson: Working as a consultant, I developed a model to predict how many registration desks a hospital would need after it switched to a new information system. I delivered a sophisticated and interactive model that allowed the end user to change a number of inputs and assumptions. But the management of the hospital didn’t have the skills or the time to understand and use the sophisticated model. I realised this only when they asked me to give them a single-page document that explained how many registration desks they needed and where.
4. There are no effective feedback loops. The data scientists’ work doesn’t end when they deliver their solution to the client or to the implementation team for roll-out across the organisation. Feedback loops are essential if you want to see continuous improvement. Feedback loops allow your team to learn as the project is in progress and continue making small tweaks that will make it more effective. Feedback loops also enable you to take lessons from that specific project to improve the next one. Sadly, most data-science projects have no process through which feedback is communicated to the data scientists. Even if such processes do exist, they may still be useless because they provide feedback on irrelevant metrics or, more simply, nobody acts on the information provided.
Learning the lesson: My team built a predictive model which was tested and found to be significantly better than what had been in place before. It was rolled out and care was taken to monitor and audit its predictive accuracy. After a while, we found that the accuracy of the model had deteriorated. No great surprise there: this often happens as the world changes and assumptions that worked well in the past are no longer valid. Nevertheless, the data science team did not have time, or indeed any incentive, to go back and tweak the model because it had moved on to other, more pressing projects. Despite the fact that feedback was available, there was no system in place to promote continuous improvement.
So how can you build a winning data science team? The most successful teams are often built from the bottom up. Top-down tends to be supply-driven: you’ve built the data science team so you’ve got to find them something to do. It is always more better to identify problems and start building solutions that are geared towards providing quick and meaningful results. Then you nurture the team to solve progressively larger problems; you then see it gain credibility and visibility within the organisation and generate enough value in terms of savings or new business opportunities to justify its existence.
Think at London Business School: fresh ideas and opinions from LBS faculty and other experts direct to your inbox
Sign up